Drone fleet control optimized by quantum technologyDrone fleet control optimized by quantum technology
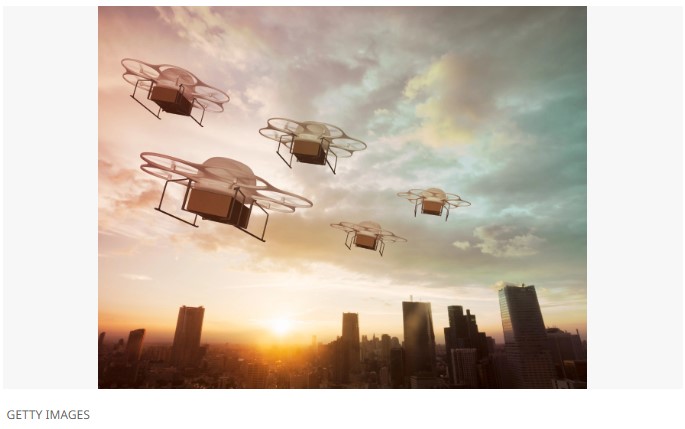
Researchers have developed a scalable hybrid quantum-classical software framework to optimize how multiple drones operate in complex, ever-changing conditions.
Drone fleets are increasingly being adopted for use cases including logistics and defense. However, their flight paths need to consider many factors that can change suddenly, like weather and other obstacles in the airspace.
A team from Korea University and Sookmyung Women’s University combined quantum and classical computing methods known as Quantum Multi-Agent Reinforcement Learning (QMDRL) to optimize multi-drone mobility.
Multi-agent reinforcement learning is used for systems where multiple agents, such as drones, must interact and adapt to evolving conditions. It uses “reward values,” numerical signals that provide feedback to a drone about how well it is performing a given task to enable better decision-making.
The complexity of coordinating this for multiple agents and adapting to dynamic environments makes scalability challenging for classical computers.
The QMDRL framework integrates quantum computing’s strengths in handling complex many-body problems with classical computing “gradient descent,” a mathematical technique used in machine learning and optimization problems.
To read the complete article, visit IoT World Today.