Generative AI’s masters are coming for your networkGenerative AI’s masters are coming for your network
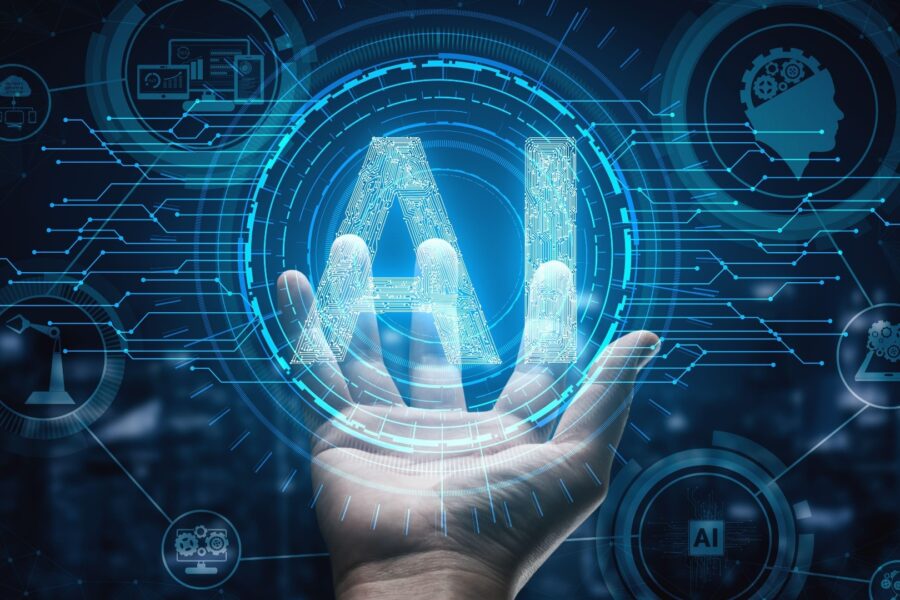
“Copilot” is suddenly a popular word to humanize “generative” artificial intelligence, the incarnation that has sparked new fears about job losses and murderous robots. It was almost a standard response to questions about genAI’s potential impact on the workforce at this year’s Digital Transformation World (DTW) event in Copenhagen. Microsoft’s code-writing genAI is even called GitHub Copilot – a truly bizarre moniker that to British ears sounds like an insult shouted in a plane.
The idea is partly to make genAI seem more of a smiling assistant than a sinister force, or at least more of a useful tool. It’s not genAI that will replace you, say the genAI salespeople. It’s the person who wields genAI when you don’t or won’t. But the copilot comments also reflect genAI’s current limitations. Prone to making stuff up, genAI could never be trusted on full autopilot. And there are arguably even greater concerns among telcos eyeing the tech.
Avoiding it is now almost impossible, judging by this year’s DTW. Frowned on by some, herd mentality is encouraged in the telecom sector, where clubs, standards and interoperability are all deemed necessary to avoid fragmentation and survive the Big Tech onslaught. A stampede in the direction of a new buzzword every few years is routine. Of course, genAI is no mere buzzword, said various executives in Copenhagen – an assertion that was made about every previous buzzword.
Speaking the right language
Perhaps the biggest concerns are about who builds, owns and supports the tech. And first off are the large language models (LLMs) themselves. The best known include the GPT (generative pre-trained transformer) range from OpenAI, the research lab that has reportedly received $10 billion in Microsoft funding. Alongside these are the wackily named Llama and Llama 2, open-source models released early this year by Meta. Others include the more conventionally christened Claude, the product of a startup called Anthropic. None of these LLMs, though, was built with telecom operations in mind.
Untrained on much telecom data in their original guise, they are of limited usefulness when it comes to the specifics. The answer is an adaptation or fine tuning to make them more telco-relevant, which could be done either by a telco or a third party such as Amdocs, a big vendor of telco software that has already part-built an LLM branded amAIz, using Microsoft and OpenAI technology. “We are not the engine,” said Gil Rosen, the chief marketing officer of Amdocs. “Basically, we are training the large language model on our telecom data set and creating our own version.”
There are many sensible reasons why Amdocs rather than a telco should do this. “Honestly, it requires more than a single telecom data set,” said Rosen. “And that is why it will only work in an alliance or coming from a company like us.”
With their obvious focus on serving customers and maintaining networks, telcos remain relatively inexperienced and unskilled in software. Building and training LLMs would gobble telco resources and put a further squeeze on profit margins already under pressure. “It is a lot of money to build an LLM,” said Danielle Royston, the CEO of Totogi, a small vendor in the market for business support systems.
But some telcos are dipping their toes into the water, with Telus one of the most outspokenly enthusiastic about in-house development. “This is a strategic imperative for us – to both understand generative AI and to have that muscle around it,” said Hesham Fahmy, the Canadian operator’s chief information officer.
Among other things, Telus has been experimenting with off-the-shelf platforms including Stability AI’s Stable Diffusion and Google’s Palm, as well as Llama and OpenAI. Using an in-house team of data scientists, it is effectively playing these models off against one another to figure out which is most suitable where. “It is less about the foundation model and the size of the model,” said Fahmy. “You can get better responses by taking a smaller model and tuning it with your own embeddings.”
Taking charge of fine tuning may hold several attractions for operators. First, handing responsibility for this to a third party would naturally increase the risk of confidential company information being used to train a model eventually deployed by rivals. At the same time, a telco with its own resources would be less reliant on any vendor for critical software and the expertise around it.
To read the complete article, visit Light Reading.